Understanding Machine Learning: What Is It and How It Transforms Business
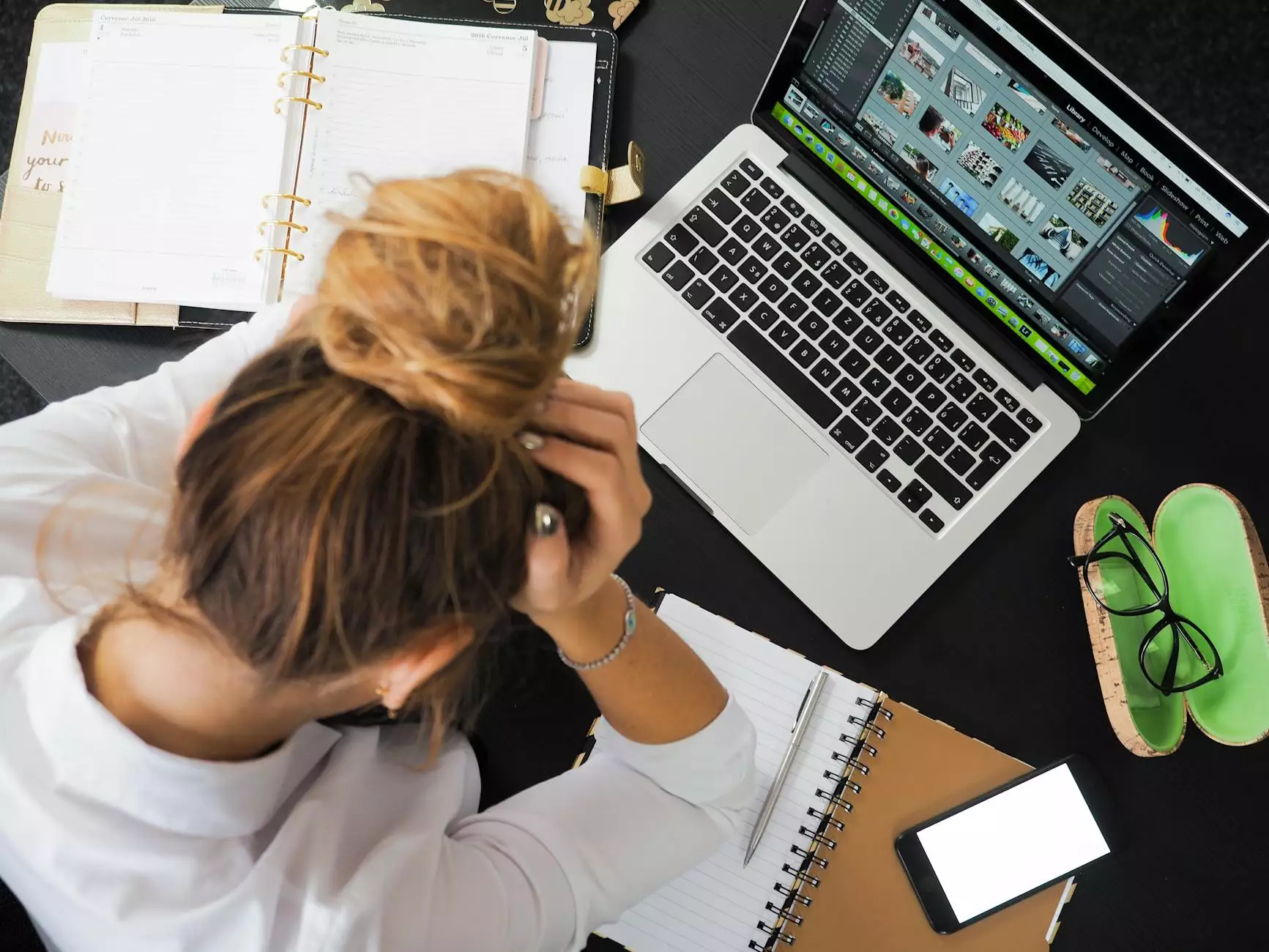
In today's digital age, the term "machine learning" is becoming omnipresent. Businesses across various sectors are beginning to grasp its potential, utilizing it to gain insights, enhance performance, and drive innovation. This article delves deep into machine learning, offering a comprehensive exploration of machine learning what is and how it's transforming the landscape of modern business.
What Is Machine Learning?
Machine Learning (ML) is a subset of artificial intelligence (AI) that focuses on the development of algorithms allowing computers to learn from and make predictions based on data. Rather than being explicitly programmed to perform a task, machines use statistical methods to identify patterns in vast amounts of data and improve their performance as they process more information.
The Evolution of Machine Learning
Historically, machine learning has roots in the field of computer science and statistics. The concept dates back to the 1950s when researchers began exploring ways for computers to learn without human intervention. Since then, advancements in computational power and data collection methods have catalyzed a boom in the capability and application of machine learning technologies.
How Does Machine Learning Work?
At its core, machine learning involves several key components that enable it to function effectively:
- Data: The foundation of machine learning is data. This can range from structured data, like databases, to unstructured data, such as images or text.
- Algorithms: These are the methods that act on the data to make computations and predictions. Common algorithms include decision trees, neural networks, and support vector machines.
- Training: In this phase, the algorithm processes the data, identifying patterns and relationships. The more data it encounters, the more accurately it can predict outcomes.
- Validation: This involves testing the trained model on new data to assess its performance and ensure it generalizes well to unseen instances.
Types of Machine Learning
Machine learning can be broadly categorized into three main types, each with distinct applications and methodologies:
1. Supervised Learning
In supervised learning, the model is trained on labeled data, where the outcomes are already known. The goal is to learn a mapping from inputs to outputs and make accurate predictions on new, unseen data. Common applications include:
- Email filtering (spam detection)
- Sentiment analysis
- Credit scoring
2. Unsupervised Learning
Unsupervised learning involves training a model on unlabeled data—meaning the outcomes are not provided. The objective here is to identify patterns or groupings within the data. Some key applications include:
- Customer segmentation
- Anomaly detection
- Market basket analysis
3. Reinforcement Learning
Reinforcement learning is a dynamic approach where an agent learns to make decisions by receiving rewards or penalties. This type of learning is commonly used in robotics, gaming, and navigation tasks.
The Impact of Machine Learning on Business
As we explore machine learning what is, it's essential to understand its profound impact on modern business practices and decision-making processes. Here are several ways in which ML is reshaping the business landscape:
1. Enhanced Decision Making
Machine learning equips businesses with the tools to parse through and analyze large datasets quickly. By revealing hidden patterns and insights, ML enhances decision-making processes and provides actionable intelligence, allowing companies to respond proactively to market changes.
2. Personalized Customer Experiences
By leveraging machine learning algorithms, businesses can analyze past customer behavior to tailor marketing messages and recommendations to individual preferences. For example, e-commerce platforms use ML to suggest products based on users' previous shopping behaviors, significantly improving user engagement and conversion rates.
3. Improved Operational Efficiency
ML can automate many business operations, leading to increased efficiency. For instance, predictive maintenance algorithms can analyze equipment performance to anticipate failures before they occur, resulting in reduced downtime and maintenance costs.
4. Risk Management and Fraud Detection
Financial institutions are increasingly adopting machine learning to manage risks and detect fraudulent activities. By analyzing transaction patterns in real-time, these systems can flag suspicious behavior faster than traditional methods, thus safeguarding assets and maintaining consumer trust.
Challenges in Machine Learning Implementation
Despite its numerous advantages, the adoption of machine learning also poses significant challenges:
- Data Quality: High-quality data is crucial for effective machine learning. Poor or biased data can lead to inaccurate models.
- Complexity: The complexity of ML algorithms can make them difficult to interpret, leading to challenges in gaining stakeholder trust.
- Skill Gap: Many organizations face a shortage of skilled professionals capable of developing and maintaining ML systems.
Future Trends in Machine Learning
As technology continues to evolve, several trends in machine learning are anticipated to shape the future of business:
1. Automation of ML Processes
With the advent of AutoML (Automated Machine Learning), organizations will increasingly automate the process of deploying machine learning models, making it more accessible to companies without extensive data science expertise.
2. Integration with Edge Computing
The combination of ML and edge computing will allow real-time data processing closer to data sources, enhancing speed and performance, especially for IoT applications.
3. Greater Focus on Ethical AI
As machine learning continues to penetrate everyday life, there will be a growing concern regarding privacy, bias, and the ethical implications of AI systems. Businesses will need to invest in frameworks that ensure responsible and transparent ML practices.
Conclusion
In summary, understanding machine learning what is and its application in business is crucial for companies looking to stay competitive in an increasingly data-driven marketplace. By leveraging the power of ML, organizations can enhance decision-making, personalize customer experiences, and improve operational efficiencies. However, as they embrace these technologies, they must also address the inherent challenges and ethical considerations that accompany them.
The insights derived from machine learning are no longer just an option but a necessity for businesses that aspire to thrive in the future. As the world moves further into the era of machine learning, understanding its fundamentals becomes imperative for any business aiming to harness its transformative potential.